Article
The 5 Fastest Growing Types of AI & How SMEs/SMBs Can Leverage Them
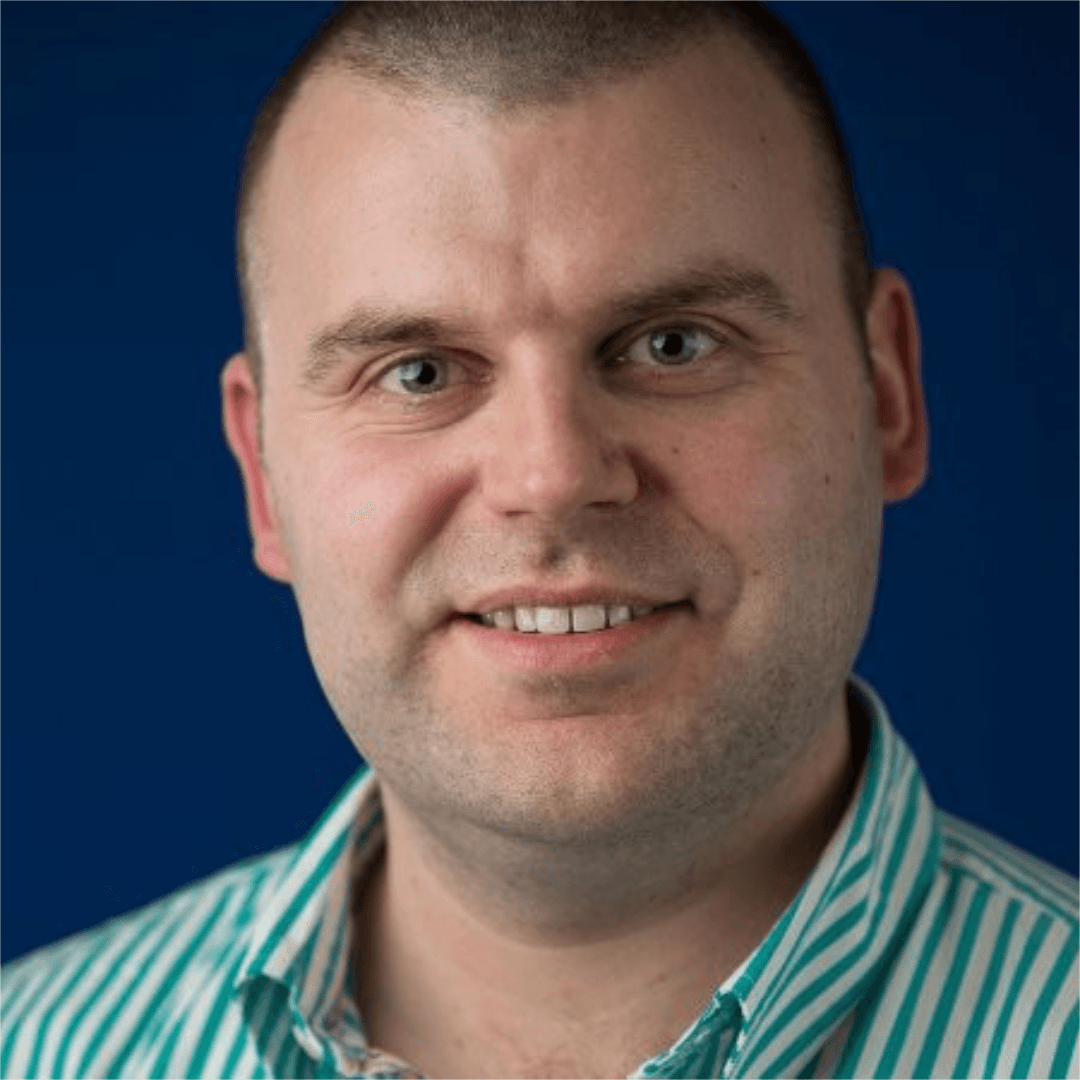
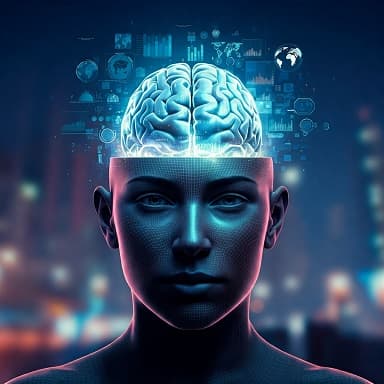
How can SMEs/SMBs leverage AI technologies such as NLP, Geospatial AI, Graph AI, Time Series AI, Computer Vision?
You Can Apply AI To Your Business
It soon became apparent that when tech jargon and mystery is stripped away, SME owners/execs are quick to see the potential applications of complex technology that once, probably, seemed daunting.
-- Graham Roberts
If you need expert tech advice to unlock growth at your business, sign up to our newsletter where we share playbooks, deep dives and peer experiences relating to tech driven growth for SMEs/SMBs.
Contents
- Intro
- What is NLP?
- What is Geospatial AI?
- What is a graph and what is Graph AI?
- What is Time Series AI?
- What is Computer Vision?
- Examples of NLP
- Examples of Geospatial AI
- Examples of Graph AI
- Examples of Time Series AI
- Examples of Computer Vision
- Wrap Up
- What Next?
Intro
The SME Growth Cohort met on 23rd October 2024 to discuss some of the fastest growing types of AI, example applications and how cohort members could potentially apply the concepts to their own businesses. It quickly became apparent that when tech jargon and mystery is stripped away, SME owners/execs can quickly see the potential applications of complex technology that once, probably, seemed daunting.
We started by listing out the 5 fastest growing problem domains that AI is solving for, according to a recent Databricks survey.
- NLP (Natural Language Processing) - 75% year on year growth
- Geospatial AI - 55% year on year growth
- Graph AI - 44% year on year growth
- Time Series AI - 42% year on year growth
- Computer Vision - 34% year on year growth
Next we did some jargon busting, but before we get into that, take a moment to join the cohort so you can start using tech and AI to drive your business forward as well.
The cohort is all about helping small business owners to adopt modern technologies, such as AI, to drive business growth. You'll hear about battle tested playbooks and you'll get to ask your questions in live Q&As at our weekly, online meetings.
It's FREE OF CHARGE until we hit 20 members.
Graham’s SME Growth Cohort has been packed full of useful takeaways, relevant to any forward-thinking business owner. As the founder of a new tech startup, it was great to be able to ask direct questions and learn from the experience of not just Graham, but the other members of the cohort too. From introductions, to tech knowledge and strategy, these sessions are an absolute must for any SME business owner that is keen to learn how to do things better than they already are. Would definitely recommend!
-- Tom Dalton, Stealth Startup
What is NLP?
NLP, or Natural Language Processing, is the understanding of language based inputs, understanding the context of what the user is asking for. Think back to the early days of Google, all the way back to circa 2001. If you did a search for "grass care business" Google didn't really understand what you were searching for, it simply looked at each keyword and retrieved documents from its database that matched those keywords. It would do some clever, algorithmic processing to rank those documents for the search engine results. It was probably looking at things like keyword density, keyword position in the document, keywords in backlinks, urls etc etc - matching the keywords was a big deal.
Fast forward to 2024, and if you do the same search, the number 1 organic result for me doesn't even have the keyword "grass" in it. Furthermore, the highlighted keywords in the results are "lawn mowing service". Now, with semantic understanding, Google can recognise that "grass care" is an alternate way of saying "lawn mowing".
So that's what NLP is, actually understanding the meaning behind a language based input.
What is Geospatial AI?
Heck, what does geospatial even mean?
Well, "geo" tells us we are talking about something relating to the earth's surface while "spatial" relates to the position, area and size of things. Therefore, geospatial is concerned with the relative position of things on earth's surface.
Geospatial AI layers in other data sources, such as satellite imagery, geographic data, demographic data etc in order to be able to make predictions about things that are location specific.
What is a graph and what is Graph AI?
A graph is a network of connected things. Those things could be:
- People, connected in a family tree
- Servers, connected in a computer network
- Towns, connected by roads
A graph is as much concerned about the relationships between data points as it is between the data points themselves. For example, is a road between towns an A road or a B road, does it have roadworks, is there a speed camera?
Graph AI brings predictive capabilities to these graphs.
What is Time Series AI?
A time series is a dataset that is indexed on time, for example, daily sales figures, monthly maximum temperatures, and intraday stock prices.
Time Series AI is concerned with predicting future values by exploring complex patterns and relationships in the historic time series data.
What is Computer Vision?
Computer vision is concerned with the interpretation and analysis of visual data. That is a huge scope of possible applications given that visual data could be of people, objects, the environment, skin tumours etc, the possibilities are endless.
With the jargon out of the way, now we are going to look at some example applications of each of these types of AI.
Examples of NLP
Chatbots have been around for ages but until not so long ago most were pretty limited. They tended to be based on a predefined logic tree driven by associating particular keywords from customers with canned responses retrieved from the logic tree. NLP has transformed this.
The logic tree now seems somewhat primitive. Instead a business can define the various contexts their chatbot should be able to handle, for example, check the delivery status of an order. The chatbot uses NLP to understand the context of the user's input. If it can match the input to one of the contexts it has been tasked with serving, it will get to work, if it cannot then most likely it will invite the customer to connect with an agent for further help.
Taking the delivery status example a step further, NLP enables the chatbot to understand not only that the customer is asking about the delivery status of their order, but also whether or not the customer has supplied their order number in their query. If not the chatbot can reply to the customer asking them to submit this data.
With the necessary information gathered the chatbot is then able to request the delivery data from the backend system, an ERP perhaps, and then respond to the customer. But let's say we have done a good job of getting to know our customers and we know that some of them like a nice, wordy answer with lots of pleasantries, while others just want the basic info given to them as succinctly as possible. Our chatbot, using an LLM (large language model), can generate a written response to the customer in the style preferred by that customer - we can automatically tailor our communications based on customer preferences. This process of "generating" a written response is behind the term "Generative AI".
In this example we used an implementation pattern known as RAG, Retrieval Augmented Generation), which allows your chatbot to respond with accurate results. If you have read about AI "hallucinating", or responding with inaccurate results, often that is because it is just generating a written response to an input it understands based on its own dataset that is mostly scraped from public sources. While this can result in perfectly readable text that sounds entirely pllausible, it is not always accurate. The RAG pattern allows you to "retrieve" trusted data from your own, private data source, such as an ERP or database, and then have the language model create the written rendering of that data, without changing any of the facts so you can rely on it being accurate.
NLP can also be used for fact extraction. This is the process of analysing unstructured data, such as a feedback form, info document, email etc, and extracting facts such as order numbers, customer satisfaction, address details etc.
A similar approach can be used to detect topics in unstructured data. For example, you could take all of your customer feedback forms and have them analysed with NLP to detect frequently occurring topics, for example, are a chunk of customers all complaining about the same thing, even if they are using different language to describe it?
Sentiment analysis is another type of NLP and this time language based inputs are analysed to assess whether those inputs are, say, good, bad or indifferent, or perhaps, satisfied or dissatisfied. Typical applications of sentiment analysis are for routing and prioritising customer services enquiries or "listening" to your social channels to ensure you respond quickly to any negative comments to protect your brand.
Examples of Geospatial AI
Town planning is being transformed by GeoAI. Planners now have easy access to information such as the number of trees along a street, land use surrounding vacant buildings in a neighbourhood, frequency of road traffic accidents etc.
Natural disaster emergency response teams are able to direct their aid efforts to minimise casualties by combing data about the likely spread of the disaster with demographic information.
Farmers can optimise their harvest yields by detecting and responding to invasive species and identifying land that appears to be less fertile in order to make appropriate and timely interventions.
Insurance companies are able to rapidly carry out impact analysis where they are exposed to a natural disaster.
The field of architecture is able to use GeoAI to help design more energy efficient buildings by making predictions about how different architectures might consume energy, for example by considering the relative position of the building to other buildings or natural features they might block sunlight.
Examples of Graph AI
Fraudulent transactions can be identified by making predictions based on a knowledge graph that includes payment transactions, people, products, address etc. AI is used to analyse a knowledge graph of historic data to understand and recognise the shape of "neighbourhoods" of connected nodes in the graph that have tended to be associated with fraudulent transactions. Then, in real time the AI is able to analyse whether new transactions appear similar to the shapes of those fraudulent "neighbourhoods", and if they do, the transaction can be flagged as likely to be fraudulent.
The impact of road closures on traffic levels can be predicted by understanding that traffic will be diverted to other roads, which might have lower capacity for vehicles. Similarly, the impact of new build housing estates on traffic levels could be modelled.
Cybersecurity uses network graphs to detect anomalous patterns of communications between devices connected via a computer network.
In drug discovery it is important to understand the interactions between genes, proteins, diseases and more, all of which can be represented using a graph. By understanding the relationships between each of those components, GraphAI is able to support pharmaceutical scientists in discovering drugs that are likely to be effective at treating certain diseases.
Examples of Time Series AI
Using statistical methods to predict future values based on historic data is not new. What AI adds to time series analysis is the ability to identify patterns in historic data to understand a dynamic process. A bit like in NLP, where the evolution was the understanding of text from simply recognising and retrieving matching keywords.
One domain to which time series AI can be applied is business sales forecasting. Using just historic sales data to forecast future sales might be overly simplistic. What impact does the macroeconomic backdrop have? What about energy prices, currency fluctuations, weather, political climate etc.
The same can be said of capacity planning - when is the best time to hire more staff or build a new warehouse?
Anomaly detection can be used to identify outliers, or odd data points in your time series. This could be a monthly sales figure. If it is way up, or down for that matter, beyond expected norms, perhaps to you need to investigate before a large problem manifests itself.
Examples of Computer Vision
There are almost countless applications of computer vision (CV), but one of the hottest areas right no is facial biometrics, in particular facial recognition and age estimation. An algorithm is able to analyse a facial image or video and create a map of facial features. By analysing those features and the relationships between those features in the facial map the computer is able to predict who somebody is, how old they are, what sex they are.
Visual query analysis (VQA) is a field of research and development that is rapidly advancing in the era of generative AI. VQA is the ability to classify an image according to the features present in the image. For example, this could be used to describe the severity of damage caused to a car in an insurance claim, to describe the condition of an item being listed for sale in an auction or to describe the colour of someone's hair in a portrait.
CV can be used for object detection. Perhaps self driving cars will use object detection to avoid harming pedestrians. Smart buildings could automatically detect fire, or detect people to control whether lights should be on or off to improve energy efficiency.
Content moderation is another form of CV that can be used to protect minors from online harms by filtering out age inappropriate imagery.
Wrap Up
Some of those themes, many perhaps, may seem quite abstract when you think about your business, but when we discussed these examples in our cohort we realised that there are many ways to use these concepts in SMBs/SMEs.
We found that local service providers could use GeoAI to plan the best towns/cities in which to operate, and to predict who is most likely to need their services and when.
We found that special interest social networks could use GraphAI to promote and accelerate community building.
We found that museums could use Computer Vision to automatically detect artworks in need of restoration before it is too late.
We found that business owners could forecast the sales, perform capacity planning, predict when plant will need replacing and model the impact of all of that on working capital requirements.
Where can your business apply AI?
What Next?
If you'd like adopt AI but don't know how or where to start, the SME Growth Cohort is ideal.
It's also free until we hit 20 members.
It is a group of SME owners/execs who share an interest in using technology to drive business growth.
We meet virtually once a week for a live Q&A and discussion around trending topics such as AI adoption.
You can ask your own questions or just listen in to soak up ideas and experiences.
I have worked with Graham since 2016 in what started as a technology advisor role. Our team quickly learned that he brought invaluable business acumen. Whether it has been technical mentorship, establishing processes, hiring freelancers, adopting AI or exploring the intersection of business and technology, Graham's input has been a critical part of unlocking our growth. Joining his Growth Cohort would be time and money well spent.
-- Tim Waxenfelter, Endurance Learning
You can also join our newsletter for more playbooks, frameworks, deep dives and peer experiences.