Article
An AI Adoption, Automation & Transformation Framework for SMEs
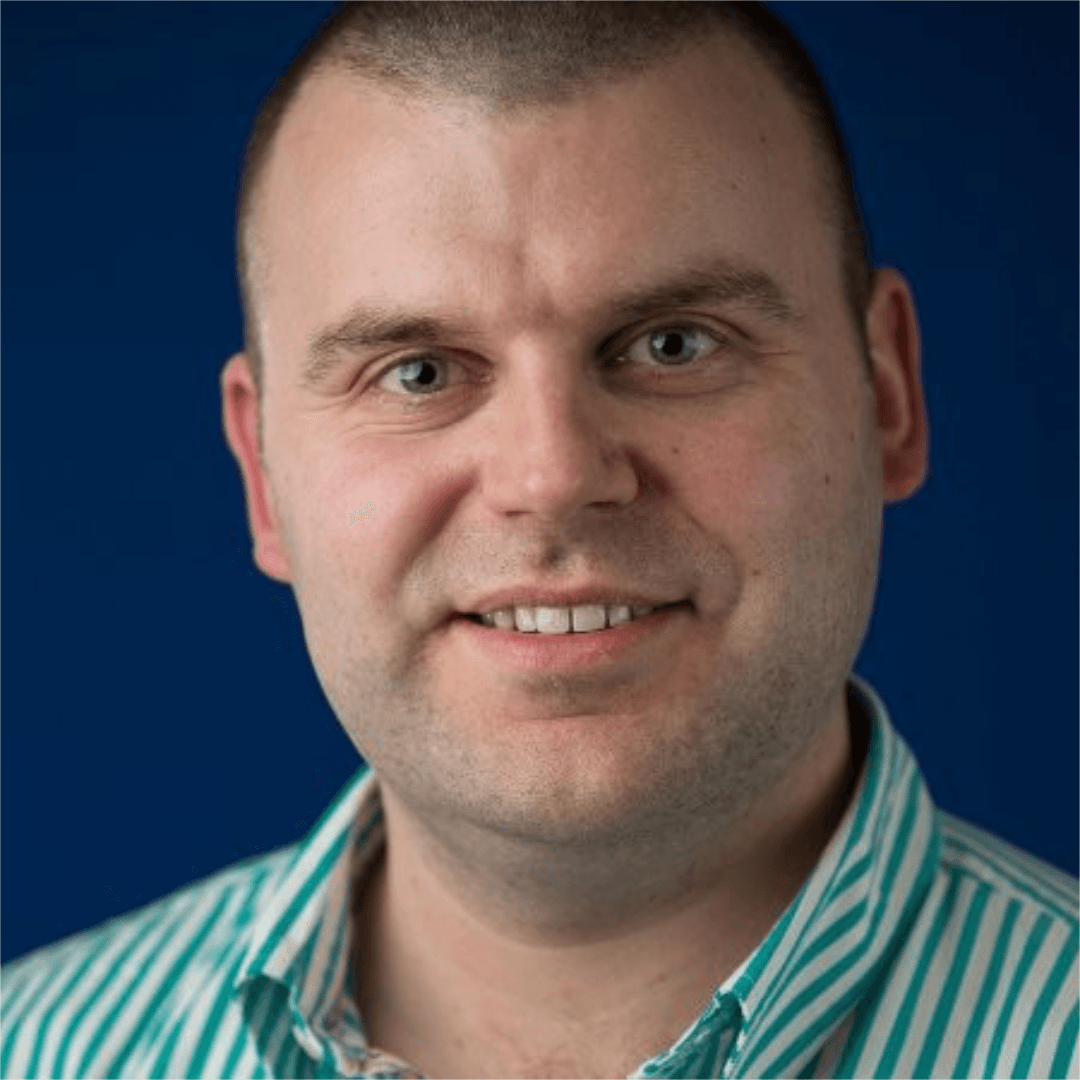
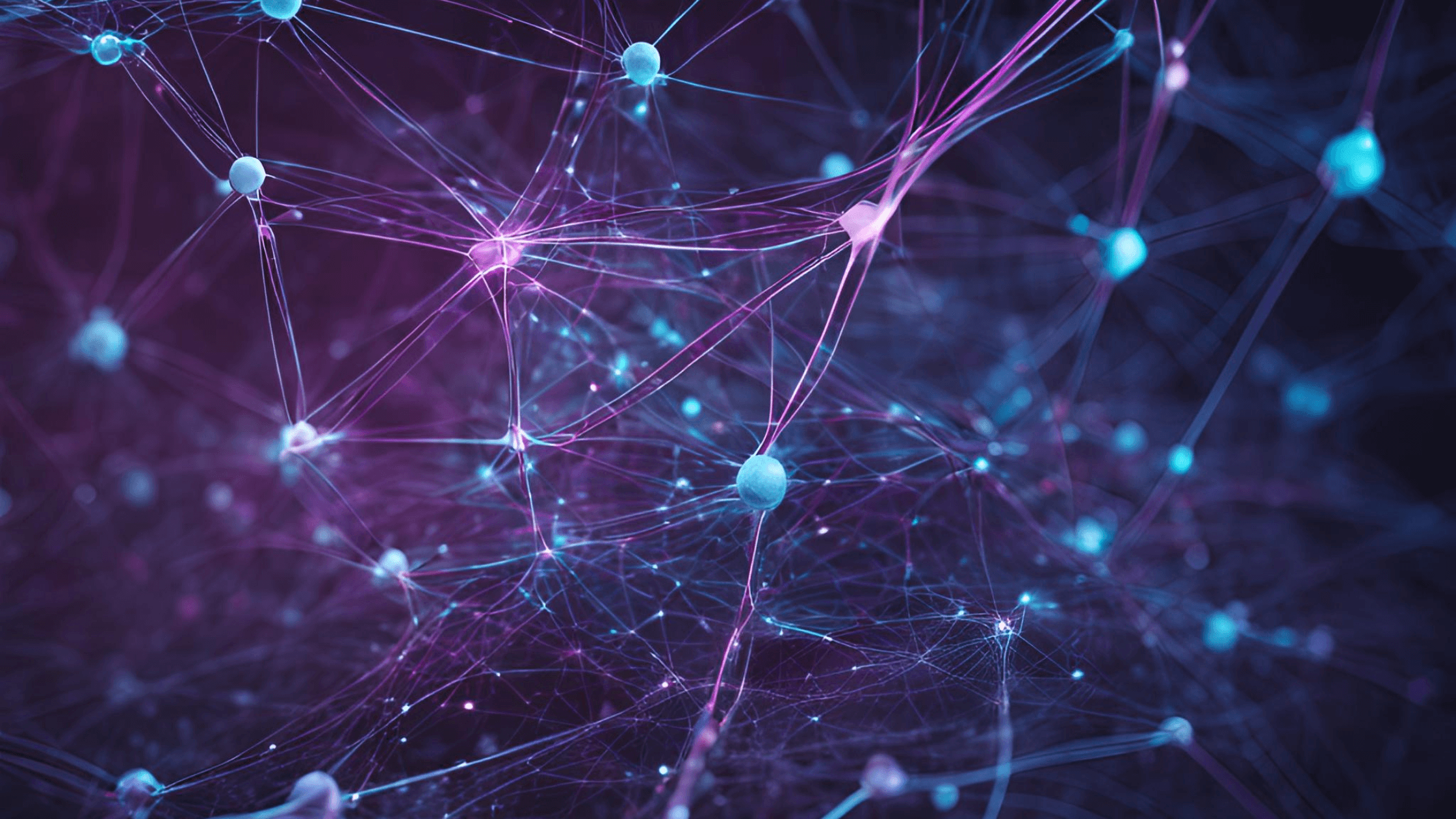
Now is the time to think about how to apply AI within your business.
Contents
- Now is the time to think about AI automation
- How to avoid AI false starts that burn cash and time
- Identifying appropriate AI solutions for your business
- Data quality, accessibility and its impact on AI success
- The skills your teams will need to develop or acquire in order to succeed with AI
- Evaluating the Costs, Benefits and ROI of AI Automation
- The Risks, Regulatory Landscape & Governance Considerations When Adopting AI Systems
- Delivering great customer experience with AI
- Wrap Up
- What Next?
Now is the time to think about AI automation
Searches for "ai" have plateaued in 2024 at approximately 70-80% of the peak volume in 2023
According to Google trends, search volume for "ai" peaked in April 2023, most likely fuelled by the excitement caused by ChatGPT's launch in November 2022. Much of this buzz can be attributed to consumers testing out this ground breaking capability to generate text, then images, then video based on simple prompts.
Many examples were truly mind blowing and the rate at which they could circulate globally via social media created a flywheel effect, generating more and more interest and demand for generative AI, which created more and more mind blowing examples to fuel growth further.
Searches for "ai" have plateaued in 2024 at approximately 70-80% of the peak volume in 2023, with monthly search volume currently around 250,000. But as this generalised hype in AI flattens off, its commercial value is starting to be realised and commercial concerns are surfacing.
Google Trends data shows that in Jan 2023 barely anyone was searching for "ai challenges", but searches for this term peaked in March 2024 with monthly search volume for this and closely related terms currently around 1,000/month. The significance of March 2024 could be the passing of the EU's AI Act, causing firms to shine a light on the risks and compliance obligations of AI adoption.
Searches for "ai automation" peaked in June 2024 before dropping, most likely as swathes of Europe shut down for summer holidays. My expectation is that this is where the action is at and that we will start to see the buzz in this area increasing from September 2024 onwards.
For that reason, this article discusses the big topics you need to be thinking about if you are considering AI Automation at your business.
How to avoid AI false starts that burn cash and time
AI is transformative.
From early diagnosis of tumours to predictive maintenance of machinery or enhanced customer experiences, AI has already changed the rules of the game.
Billions of Pounds and Dollars and Euros and Yuans are poured into AI pioneers who maintain a remarkable pace of innovation.
However, it isn't the same for those SMEs who wonder whether they should adopt AI, where it makes sense and how on earth to go about it.
We get this with every tech shift: cloud, big data, blockchain, etc. As SME owners/execs hear about pioneering companies driving top line growth and margin expansion, they want in on it.
But the failure rate of companies adopting these technologies tends to be high. Unfortunately, when the commercial goal is "adopt tech X", you are almost guaranteed to fail. Far better to set a commercial goal such as "enhance operating efficiency by 5%" or "increase customer lifetime value by 10%" and then assess what tech gets you there.
The first rule for avoiding false starts is: begin by examining your commercial goals, challenges and opportunities.
It's worth thinking about your AI readiness. AI provides most utility when it has plenty of high quality data. If your data resembles a bin fire then you'll want to sort that out, otherwise your AI solutions are likely to be severely hindered.
People are an important factor. Most AI systems need human operation or oversight. How will you reskill your team to use these tools? What about your customers? Will your target demographic embrace change?
A great way to set fire to your cash, just as good as rule #1, is to allow the solution to your commercial goal to be overly focussed on the tech, rather than the commercial outcome you should be optimising for. Sometimes this happens when the perceived value of the IP you'll create is enormous. But remember, that IP is worthless if it doesn't work.
Take a fictitious insurance company with a commercial goal of improving customer retention by enhancing CSAT, to be achieved by speeding up insurance claim handling by 75%, which is achievable by deploying an AI copilot to the claims team. The company could hire a team of AI engineers to build an insurance based LLM and accompanying copilot. However, the outcome is likely to be wasted money, inferior accuracy, a dejected team and loss of trust in AI initiatives.
On the other hand, you could look for AI models offering a solid starting point in terms of data privacy, accuracy, ability to be fine tuned etc, meaning you could deliver a high impact, high value tool with a strong ROI for the business.
No, you won't be an AI company, but you will get the actual benefit of being an AI enabled company.
The adage is true, don't reinvent the wheel. A vast array of AI building blocks can be used to assemble hugely valuable systems for a fraction of the cost of doing it yourself and with a much greater chance of success.
Identifying appropriate AI solutions for your business
If we should avoid burning our SME's cash and time on wasteful AI projects by starting with an assessment of our commercial goals, challenges and opportunities, how do we then identify appropriate AI solutions to those goals, challenges and opportunities?
For inspiration, Databricks' 2024 State of Data + AI report lists the fastest growing problem domains that AI is solving for as:
- NLP (Natural Language Processing) - 75%
- Geospatial - 55%
- Graph - 44%
- Time Series - 42%
- Computer Vision - 34%
Since November 2022 most of us have used NLP, even if only experimenting with ChatGPT. It is at the heart of the generative AI technologies that power chatbots around the globe. But, increasingly SMEs are deploying NLP behind the scenes. Not for its generative capability, but for its ability to understand language based inputs, extract facts, enrich data, and enable end to end automation.
Geospatial AI has use cases ranging from city planning to maximising farming yields, assessing insurance risks and beyond. What these disparate industries have in common are outputs that are impacted by spatial data, location data, satellite imagery and other forms of geographic data. Geospatial AI models are capable of analysing these industries' geospatial attributes to create predictive power in explaining population growth, identifying poor crop health and measuring insurance risk, and much more.
Graph theory is concerned with connections between nodes. This could be connections between people in a social media graph, computers on a network or even road networks connecting towns. Graph AI brings predictive capabilities to these graphs. For example, examining the connections between online payments, webshops, people, addresses and more to predict whether a transaction is likely to be fraudulent, or even whether the customer is likely to be interested in a particular upsell.
Time series AI examines historical data points and looks for patterns that can be used to predict future events. This could be a super complex model that takes account of many variables, such as in weather forecasting, or it could be something more relatable to SMEs, such as sales forecasting or capacity planning.
Computer vision AI has a huge array of applications from facial biometrics, tumour diagnosis, fire detection, document processing or even damage evaluation in car insurance claims. Any business that processes visual inputs could potentially streamline their operations and increase their accuracy using AI models that are trained to evaluate images and turn them into actionable data.
There are other types of AI as well, but the key is to recognise that they each tend to offer either:
- the ability to predict events/outcomes
- the ability to classify things
- the ability to detect anomalies
If your business could be enhanced with any of those capabilities, AI could be an appropriate solution.
Data quality, accessibility and its impact on AI success
Imagine that data quality is low at your business: customer records are incomplete or duplicated, inventory data tells a different story to warehouse staff and your financials have journal entries all over the place to make the books balance.
How much do you rely on that data for big decisions?
Will you just do what the data says?
Will you pass your data through a pipeline of Excel spreadies to fix, check and double check your data?
Or, will you ignore your data, knowing that at best it's unhelpful and at worst quite damaging to your business?
You can't fix that by parachuting some AI into your business.
An AI system would make predictions that are unhelpful, since they would be based on unreliable data, just as in the human example.
It could not classify a good from bad customer because, quite frankly, those duplicate records and incomplete data might make this impossible to determine.
And it might miss a hot sales streak due to bad inventory data meaning you didn't automatically restock in time meaning potential sales are lost.
If data problems would hinder a human it is likely they will also hinder an AI system.
Once your data is cleaned up your AI systems also need access to it in a timely fashion.
Eg, take an ecomm business that would like to deflect 80% of post sale customer service enquiries to a bot. Canned responses based on a decision tree are not going to be sufficient. The bot needs access to customer, order and delivery data, at a minimum.
When do you think customers will start asking questions about their order?
One day later?
Two?
No, straight away.
Whether it is to check a delivery time, report an ordering error, ask about a payment glitch etc, some customers make contact right after ordering. To hit an 80% deflection rate you'll need to be handling these.
Therefore it is no good relying on a daily batch job to fetch a load of data from various systems and place it in a database that your AI system can read. You need to be thinking about near real time data so your AI bot has data it can trust when it needs it.
Ignoring data quality and accessibility issues before implementing an AI system means you are likely to encounter one or more of the following:
- Wasted time and money as your AI project cannot be put into production
- Spurious business decisions because predictions were based on flawed data
- Reputational damage because customer experience is poor
The good news is, all of data issues pre-date AI and there are well established tools and techniques to deal with them.
Your people can implement a data governance framework.
You can "shift left" your data quality, aiming for accurate data at source rather than fixing and checking it downstream.
Your systems can be integrated using iPaaS, event streams, ETL/ELT tools or similar.
The skills your teams will need to develop or acquire in order to succeed with AI
It's often reported that AI will be a big destroyer of jobs.
I don't buy it.
The ATM was going to cost bank tellers their jobs. It enabled branch openings due to cost savings. Bank teller employment increased and they became focussed on higher value tasks.
I see that story repeating with AI.
Products, services and entire industries will thrive, enabled by AI systems that alter the art of the possible. Commoditised diagnosis and treatment will transform healthcare, personalised learning experiences will transform education, real time analysis of data and market trends will transform business decision making.
But those transformations can't happen without people.
Lots of them.
AI systems are developed by scientists, engineers and researchers who are hired in their thousands by the likes of Microsoft, OpenAI, Google etc.
Your business probably needs different AI skills.
For most SMEs it won't make sense to build an AI system from the ground up, they will either:
- Procure an AI system that is designed for a specific purpose
- Refine an AI model that has been proven to work for similar use cases
Those businesses will need to build the following skills to succeed with AI:
- AI Fundamentals - make sure your business solves the right problems with AI. Spread the knowledge. Train core teams on AI basics so they know what is possible and feel empowered to identify opportunities for AI.
- Data - your teams should be skilled at collecting, cleaning, enriching, analysing and integrating data to produce high quality, accessible data sets. To some this will feel like a sideshow, but it's usually a big determinant of success.
- AI Operators - your teams should have the skills to operate AI systems. Generative AI requires prompt engineering, co-pilots require human inputs and decisions, automated decision making systems require human oversight.
- Fine tuning - if refining an AI model for your use case is appropriate you will need to build your software development capability.
- Infrastructure - unless you are buying AIaaS (AI as a service) you may need to build out an MLOps discipline for AI workflows and releases.
- Ethics - understand how your AI systems make decisions to assess ethical impacts, such as discrimination, that could cause reputational damage or fines.
- Legal - AI brings challenges ranging from intellectual property to regulatory compliance. Be on the right side of them to avoid costly mistakes.
That's a long list of skills. There are several ways to add them to your team:
- Training - many of the big AI vendors have free training materials and many commercial courses are available.
- Hiring - a lot of people are upskilling for AI roles, but there is a lot of competition for the best talent
- Outsourcing - the AI landscape is evolving at great pace. Specialist agencies are well placed to give up to date, tested advice and opinions.
Evaluating the Costs, Benefits and ROI of AI Automation
Having confirmed AI automation as the best solution for your business problem/opportunity, ensure implementing it will create business value.
It's not a given.
There are many potential costs when implementing AI. You need to validate that they are outweighed by the benefits. You need to ensure that the ROI promised by AI adoption is more attractive than alternate investment opportunities.
How?
Start with the business context.
What does your AI solution need to do to successfully solve the business requirement? What is the functional output? What is the required accuracy? What data does it need? What about security? How will people interact with it? Etc.
Asking these questions helps you form a requirements list. Pass the list around stakeholders, especially those involved with operationalising the system. The list will grow. Pass it round again. It will grow.
After a few rounds you'll have a detailed list. It will be too long. There will be wishlist items that you don't need. Not for day one. Prune the list. Pass it round. Ask what is essential, what is not.
Repeat.
Once you have stable requirements it's time to estimate.
Estimates, by definition, are going to be wrong. You are estimating with incomplete information. But I'd rather have an incorrect estimate of the capital expenditure required by an AI transformation based on a rigorous understanding of system requirements plus educated assumptions than a rudderless money pit that will likely never get delivered.
Most items on your list will relate to development costs, but AI automation typically incurs costs in several places:
- Research
- Design
- Development
- Infrastructure
- Data quality/accessibility
- Model training/fine tuning
- Testing
- Deployment
- Monitoring
- Maintenance
- Change management
Cost everything.
Next you need to put a value on the benefit AI will bring to your business. There are several areas - look beyond the obvious:
- Time saved
- Improved accuracy
- Enhanced customer experience
- Customer acquisition costs
- Customer lifetime value
- Superior decision making
- Revenue generation
- Higher staff satisfaction
With estimates of cost and benefits you can work out ROI, but a simple percentage isn't optimal. Consider using net present value or free cash flows to respect the time value of money. This considers not only the size of the costs and benefits, but also when they are realised, which can dramatically impact the attractiveness of an investment.
You also need to consider the risks.
How likely is it that the project succeeds? Is it an all or nothing bet? Are there degrees of success? Have peers succeeded with similar projects? Is your brand reputation at risk? Can you control the risks or are they external/unknown?
My rule of thumb is that the larger the risk, or unknowns, the smaller the investment I want to make and the quicker I want to be able to test ideas. Once something starts to stick we can increase our position as the risk to realising value reduces.
The Risks, Regulatory Landscape & Governance Considerations When Adopting AI Systems
AI systems have the potential to transform businesses and entire industries, but this doesn't come without risk.
AI brings a new set of risks for businesses to manage:
- Intellectual property - this is multi faceted. If you use Generative AI, do you own what you create? We aren't just talking about written text, images and video, what about the code base for your software system that was written with the help of AI?
- Cybersecurity - might a prompt injection attack cause your LLM to create harmful outputs? Could your training data be poisoned to create harmful or biassed outcomes?
- Privacy - if models are trained on sensitive data, might you leak commercial secrets? What if you are using third party services, might you be putting commercially sensitive information into the public domain?
- Bias and discrimination - how do you ensure your AI system does not perpetuate and systematise human biases that can cause brand damage and even lead to fines?
- Safety and security - AI can be predictive but it is not designed to be 100% accurate. If people will act on your AI generated instructions, how do you know those instructions are guaranteed to be safe and not based on hallucinations?
- Job displacement - jobs are already being displaced by AI and that will cause unease and anxiety across businesses. How do you avoid blowing up your company culture and alienating your team?
- Failure - there are many examples of AI systems showing great promise but never quite managing to achieve the desired level of accuracy to be operationalised, causing wasted time and money.
- Transparency and explainability - AI models might produce outputs based on billions of parameters. When those models make questionable predictions or decisions, how will you understand why to use that information to improve future outcomes?
- Human-machine interactions - a train of thought says that augmenting humans with AI to complete business processes reduces risks, another says that humans will simply do what the AI system says. In that scenario the overall risk would depend on whether the AI system is more accurate than the human, and if it is, we are back to job displacement.
The regulatory landscape is new, but evolving rapidly:
- EU AI Act came into force on 1st August 2024
- California's AI Safety Bill, SB 1047, passed on 28th August 2024
- The UK waits to see whether the new government will shift from the current context focussed approach
AI governance is an emerging discipline that coordinates with many stakeholders to tackle the risks and regulatory requirements associated with AI systems. AI governance is wide reaching and is tasked with ensuring that AI systems are accurate, fair, standards compliant, ethical, privacy preserving and trustable.
There is a lot to consider, but don't let that block your AI adoption, just plan accordingly and seek advice where needed.
Delivering great customer experience with AI
Customers are the lifeblood of business.
It makes total sense to explore how AI can enhance their customer experience.
So, what does a customer care about that can be enhanced with AI?
The main customer needs include:
- Functionality
- Quality
- Speed
- Price
Opportunities for enhancing functionality with AI are so broad that there are almost limitless opportunities, we'll consider just a few.
AI co-pilots offer foreground functionality. Staff offload work directly to their AI co-pilot ranging from drafting emails, summarising documents, taking notes in virtual meetings, editing videos and generating code for software applications.
AI can also make functional enhancements in the background. For example, retail checkout experiences can be automated with biometric age analysis and farmers can increase crop yields using predictive AI to manage farmland.
Not all functionality enhancements need to be super complex or ground breaking. Most businesses communicate with their customers periodically, often poorly. Imagine, instead of a monthly newsletter where the only personalisation is the salutation, a newsletter with:
- Delivery that is timed to maximise engagement
- Content that is tailored based on customer interactions, declared preferences and peers
- Copy that is generated in a particular tone of voice that is matched to customer preferences
You don't need a team of AI scientists to deliver this. The technology exists and is ready to be integrated into your stack.
So what about quality? The bad news is that AI isn't designed to be 100% accurate, all of the time. That sounds like quite a constraint, if we are trying to optimise for quality. But in fact, it usually isn't.
What is the alternative?
Often the alternative is a human performing a task. Humans are prone to mistakes, whether it is a typo, a tiredness induced mistake, a logic error, subconscious bias or something else.
Most processes operated by humans need to tolerate mistakes.
While GenAI systems hallucinate and have biases, they don't get tired or distracted and they don't forget to follow the rules laid down for them. The point being, if we can accept that AI can't guarantee 100% accuracy, we can often find a way for it to outperform its human counterpart.
AI best serves the need for speed via automation. This impacts every step of the customer journey: augmenting discovery with recommendation, offering 24/7 personalised onboarding guidance, monitoring customer sentiment to enable preemptive interventions, presenting next best actions on customers' preferred channels to minimise friction for subsequent purchases.
Where those AI automations enable you to shift from linear revenue growth that is correlated with headcount growth, towards exponential scale up facilitated by automated processes, it is likely that your unit economics will improve, and that will give you freedom to optimise your pricing.
Wrap Up
AI can be a game changer for many SMEs.
There is a lot to consider and new skills are required, but don't let that get in your way.
If you haven't started to experiment with AI at your SME yet, you could be losing ground to your competition, and once you slip behind it can be difficult to catch up.
Look at your key business challenges and opportunities, identify the most rewarding applications of AI and plan how to start small then double down on successes.
What Next?
We have been fortunate to work on several AI transformations for SMEs. Get in touch today for a no strings chat about how your business can unlock growth by adopting AI.
Read more about our AI transformation consulting and fractional CTO service to understand how we help clients like you.